多輸出決策樹迴歸#
一個說明決策樹多輸出迴歸的示例。
決策樹 (decision trees) 用於在給定單一基礎特徵的情況下,同時預測圓形的雜訊 x 和 y 觀測值。 因此,它學習逼近圓形的局部線性迴歸。
我們可以看到,如果樹的最大深度(由 max_depth
參數控制)設置得太高,決策樹會學習訓練數據中過於精細的細節並從雜訊中學習,即它們過度擬合。
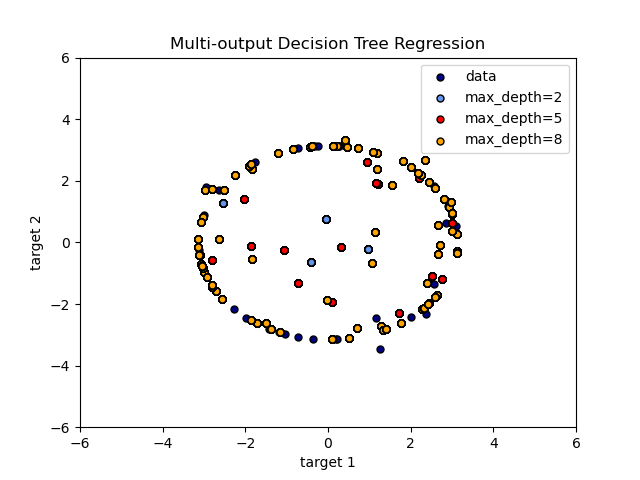
import matplotlib.pyplot as plt
import numpy as np
from sklearn.tree import DecisionTreeRegressor
# Create a random dataset
rng = np.random.RandomState(1)
X = np.sort(200 * rng.rand(100, 1) - 100, axis=0)
y = np.array([np.pi * np.sin(X).ravel(), np.pi * np.cos(X).ravel()]).T
y[::5, :] += 0.5 - rng.rand(20, 2)
# Fit regression model
regr_1 = DecisionTreeRegressor(max_depth=2)
regr_2 = DecisionTreeRegressor(max_depth=5)
regr_3 = DecisionTreeRegressor(max_depth=8)
regr_1.fit(X, y)
regr_2.fit(X, y)
regr_3.fit(X, y)
# Predict
X_test = np.arange(-100.0, 100.0, 0.01)[:, np.newaxis]
y_1 = regr_1.predict(X_test)
y_2 = regr_2.predict(X_test)
y_3 = regr_3.predict(X_test)
# Plot the results
plt.figure()
s = 25
plt.scatter(y[:, 0], y[:, 1], c="navy", s=s, edgecolor="black", label="data")
plt.scatter(
y_1[:, 0],
y_1[:, 1],
c="cornflowerblue",
s=s,
edgecolor="black",
label="max_depth=2",
)
plt.scatter(y_2[:, 0], y_2[:, 1], c="red", s=s, edgecolor="black", label="max_depth=5")
plt.scatter(
y_3[:, 0], y_3[:, 1], c="orange", s=s, edgecolor="black", label="max_depth=8"
)
plt.xlim([-6, 6])
plt.ylim([-6, 6])
plt.xlabel("target 1")
plt.ylabel("target 2")
plt.title("Multi-output Decision Tree Regression")
plt.legend(loc="best")
plt.show()
**腳本總運行時間:**(0 分鐘 0.281 秒)
相關範例